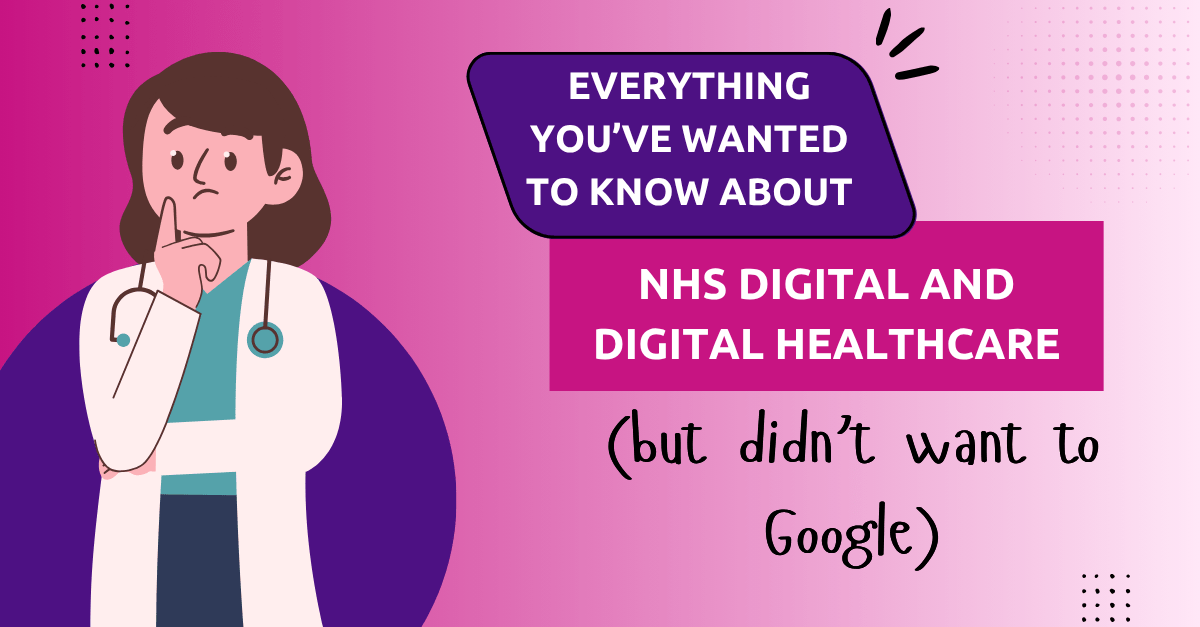
Everything you’ve wanted to know about NHS Digital and Digital Healthcare (but didn’t want to Google)
Digital healthcare can feel overwhelming – full of jargon, systems and endless acronyms. This blog breaks it all down in plain English. From what NHS digital actually does – to how apps, data tools and integrated records work behind the scenes, we explore the real impact of digital transformation in UK healthcare. Whether you’re a curious patient or a healthcare professional, this is your go-to guide for understanding how NHS technology is shaping the future of care, and what it means for you. Download the full PDF or dive in online.